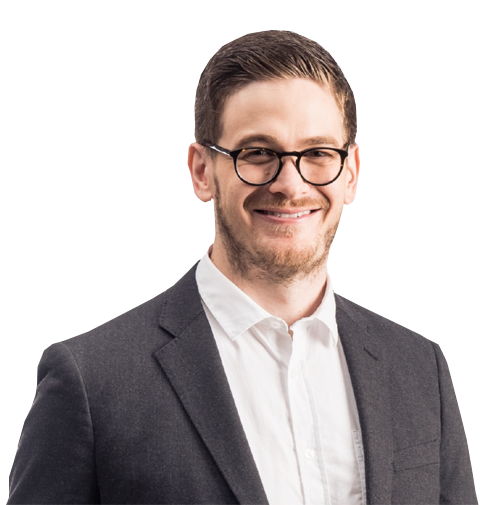
Jason PITT
My laboratory seeks to understand how patterns of somatic mutations arise within the DNA of cells. The ongoing accumulation of mutations – known as genome instability (GI) – represent a history of aberrant DNA damage and repair processes within malignancies. We combine large-scale sequencing datasets, artificial intelligence, and data-intensive computing to define and biologically characterize multi-modal GI patterns. This enables us to decipher how mutational processes fuel cancer evolution and can be exploited therapeutically.
jason.j.pitt[at]nus.edu.sg
Principal Investigator, Cancer Science Institute of Singapore, NUS
Member, NUS Artificial Intelligence Institute (NAII), NUS
2016 | Charles J. Epstein Trainee Award for Excellence in Human Genetics Research – Finalist (American Society of Human Genetics) |
2012 | Partners in International Research (PIRE) Fellow (National Science Foundation) |
2011-2014 | Genetics and Regulation Training Grant recipient (University of Chicago) |
2009 | Rupert Anderson Award for Research (Gustavus Adolphus College) |
2008 | Presidential Research Grant (Gustavus Adolphus College) |
2007-2008 | Mayo Clinic Scholars Program |
Genome instability (GI) is a hallmark cancer that drives both tumorigenesis and evolution. Despite its ubiquitous nature, we have limited understanding of GI’s origins, heterogeneity, and clinical implications. Mutations underlying GI arise from an admixture of exogenous and endogenous factors – each generating specific lesions across mutational modalities (e.g. single base substitutions [SBS], structural variations [SVs], etc.). The relative contributions of these factors can vary temporally and by tissue. This high combinatorial complexity makes comprehensive identification of distinct GI patterns intractable experimentally. Instead, the presence, activities, and sources of GI patterns can be derived computationally – particularly from vast collections of cancer genomes. Doing so highlights dysregulated mutational processes throughout a malignancy’s life history. Disentangling these processes and their interactions provides critical information on how cancers develop, can be treated, and can be prevented.
Within the Pitt Lab, we leverage big data genomics and artificial intelligence (AI) to identify GI patterns pan-cancer along with their causes, consequences, and context-dependencies. We approach this problem in multiple unique ways: i) by deriving multimodal GI patterns through innovative learning methods; ii) by developing generative AI models to predict these patterns under specific conditions (e.g. tissue, DNA repair deficiencies, etc.); and iii) with experimental validation of these predictions through synergistic collaborations. Together, these approaches address three thematic questions:
- What are the multimodal GI patterns that emerge on cancer genomes?
- What do these patterns tell us about cancer’s evolution and heterogeneity?
- Can these patterns guide cancer treatment and prevention?
- L. R. Kong, K. Gupta, A. J. Wu, … C. Frezza, J. J. Pitt, A. Venkitaraman. A glycolytic metabolite bypasses “two-hit” tumor suppression by BRCA2. Cell. 187, 1-19 (2024).
- A. Perera, V. Warrier, S. Sundararaman, Y. Hsiao, S. Ghosh, L. Kularatnaajah, J. J. Pitt¶. Melvin is a conversational voice interface for cancer genomics data. Communications Biology. 7(30), (2024).
- A. Wu., A. Perera, L. Kularatnaajah, A. Korsakova, J. J. Pitt¶. Mutational signature assignment heterogeneity is widespread and can be addressed by ensemble approaches. Briefings in Bioinformatics. 24(6), bbad331 (2023).
- M. M. Hoppe,... J. J. Pitt… A. D. Jeyasekharan. Patterns of oncogene coexpression at single-cell resolution influence survival in lymphoma. Cancer Discovery. 13(5), 1144-63 (2023).
- C. M. M. Wai, S. Chen, T. Phyu, S. Fan,. … C. Tripodo, J. J. Pitt¶, S.-B. Ng, Immune pathway upregulation and lower genomic instability distinguish EBV-positive nodal T/NK-cell lymphoma from ENKTL and PTCL-NOS. Haematologica. 107(8), 1864-79 (2022).
- J. J. Chan, B. Zhang, X. H. Chew,… J.J. Pitt, … P. K. H. Chow, H. Yang, Y. Tay, Pan-cancer, pervasive upregulation of 3’UTR splicing drives tumorigenesis. Nature Cell Biology, 24(6), 928-39 (2022).
- S. Wang, J. J. Pitt*, Y. Zheng, … K. P. White, D. Huo, O. I. Olopade, Germline variants and somatic mutation signatures of breast cancer across populations of African and European ancestry in the US and Nigeria. International Journal of Cancer. 145, 3321–3333 (2019).
- C. Wu, T. Summer, Z. Li, A. Woodard, … K. Chard, J. Pitt, I. Foster, ParaOpt: Automated Application Parameterization and Optimization for the Cloud. IEEE International Conference on Cloud Computing Technology and Science (CloudCom). 255-262 (2019).
- J. J. Pitt*, M. Riester, Y. Zheng, T. F. Yoshimatsu, A. Sanni, O. Oluwasola, A. Veloso, E. Labrot, S. Wang, A. Odetunde, Characterization of Nigerian breast cancer reveals prevalent homologous recombination deficiency and aggressive molecular features. Nature Communications. 9, 4181 (2018).
- J. J. Pitt*, Y. Zheng, O. I. Olopade, Genetic Ancestry May Influence the Evolutionary Trajectory of Cancers. Cancer Cell. 34 (2018), pp. 529–530.
* denotes first authorship; ¶ denotes corresponding authorship