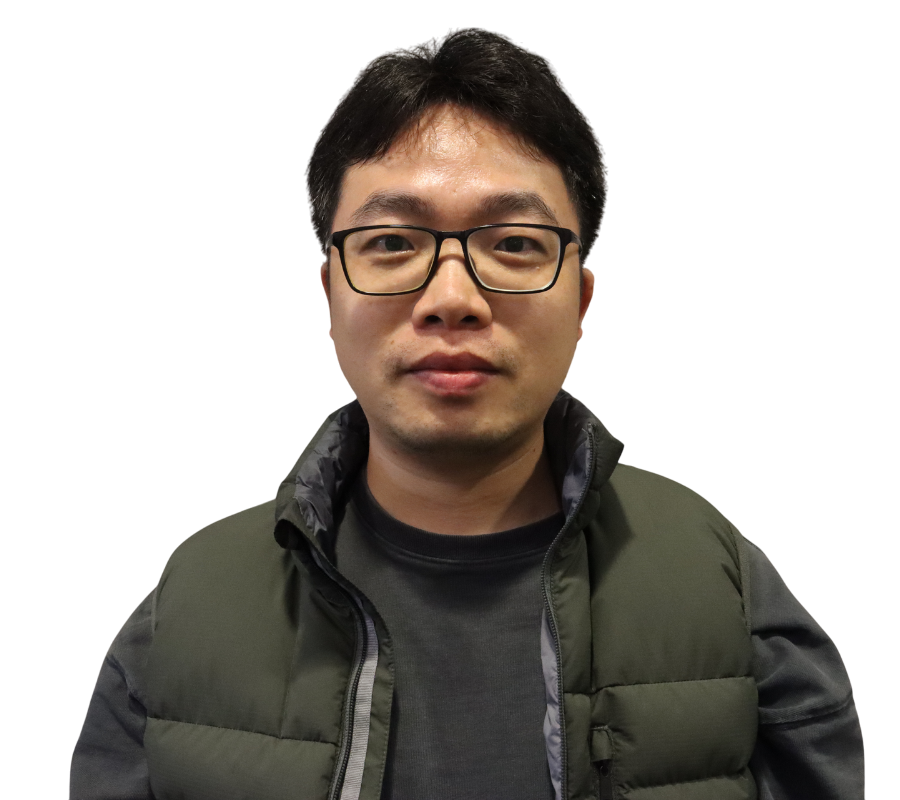
Yang Li
RNA plays critical roles in diverse cellular processes, from gene regulation to enzymatic activity. Accurate RNA structure prediction is essential to understand its biological function. The integration of co-evolutionary analysis with advanced deep learning approaches can help us predict better RNA structures. Beyond prediction, RNA design enables us to create RNA molecules with desired properties. Our lab focuses on both RNA structure prediction and design through these complementary computational approaches, aiming to unlock the full potential of RNA molecules in medicine and biotechnology.
liyangum@nus.edu.sg
Special Fellow, Cancer Science Institute of Singapore
–
We have been focusing on protein and RNA structure prediction and design through advanced deep learning approaches. More specifically, we are interested in:
1. Co-evolution analysis and learning Co-evolution analysis aims to model interactions between residues in an unsupervised manner. Initially, scientists employed statistical physics-based approaches, such as the Potts model, to analyze these interactions. More recently, language models have been introduced to capture non-linear relationships and learn interactions across multiple systems. We believe that the application of language models in co-evolution analysis remains largely underexplored, especially when trying to make connections between alignments, attentions, and contacts. Moreover, co-evolution analysis has consistently proven very useful in protein structure prediction and design. It is an exciting research area that can help us understand biomolecules at different levels.
2. Biomolecule structure prediction Protein and RNA structure prediction has long been a hot topic in computational biology. With AlphaFold series models, many challenges in this domain appear to have been addressed, at least for protein monomer structures. However, significant opportunities remain in modeling complex structures. Currently, the accuracy and performance of complex structure predictions are still limited. Co-evolution should be another key factor in modeling complex structures. Our focus is in understanding intermolecular co-evolution and modelling the detailed interactions between biomolecules.
3. Conditional sequence and structure design Meanwhile, molecular structure and sequence design represent another promising direction, particularly with the advancements in AlphaFold-based structural modeling. Researchers can now quickly evaluate the structural feasibility of their designs. Even more exciting is the potential to transition these designed molecules into practical applications, which is also a major focus of our lab and represents an exciting frontier in computational biology.
- Zheng, W., Wuyun, Q., Li, Y., Zhang, C., Freddolino, P. L., & Zhang, Y. (2024). Improving deep learning protein monomer and complex structure prediction using DeepMSA2 with huge metagenomics data. Nature Methods, 21(2), 279-289.
- Li, Y., Zhang, C., Zhang, X., & Zhang, Y. (2024). TCRfinder: Improved TCR virtual screening for novel antigenic peptides with tailored language models. bioRxiv, 2024-06.
- Li, Y., Zhang, C., Feng, C., Pearce, R., Lydia Freddolino, P., & Zhang, Y. (2023). Integrating end-to-end learning with deep geometrical potentials for ab initio RNA structure prediction. Nature Communications, 14(1), 5745.
- Li, Y., Liu, Y., & Yu, D. J. (2023). Machine learning for protein inter-residue interaction prediction. In Machine Learning in Bioinformatics of Protein Sequences: Algorithms, Databases and Resources for Modern Protein Bioinformatics (pp. 183-203).
- Zhou, X., Zheng, W., Li, Y., Pearce, R., Zhang, C., Bell, E. W., ... & Zhang, Y. (2022). I-TASSER-MTD: a deep-learning-based platform for multi-domain protein structure and function prediction. Nature Protocols, 17(10), 2326-2353.
- Li, Y., Zhang, C., Yu, D. J., & Zhang, Y. (2022). Deep learning geometrical potential for high-accuracy ab initio protein structure prediction. Iscience, 25(6)
- Zhou X, Li Y, Zhang C, et al. Progressive assembly of multi-domain protein structures from cryo-EM density maps[J]. Nature computational science, 2022, 2(4): 265-275
- Li, Y., Zhang, C., Bell, E. W., Zheng, W., Zhou, X., Yu, D. J., & Zhang, Y. (2021). Deducing high-accuracy protein contact-maps from a triplet of coevolutionary matrices through deep residual convolutional networks. PLoS computational biology, 17(3), e1008865
- Li, Y., Zhang, C., Zheng, W., Zhou, X., Bell, E. W., Yu, D. J., & Zhang, Y. (2021). Protein inter‐residue contact and distance prediction by coupling complementary coevolution features with deep residual networks in CASP14. Proteins: Structure, Function, and Bioinformatics, 89(12), 1911-1921
- Li, Y., Hu, J., Zhang, C., Yu, D. J., & Zhang, Y. (2019). ResPRE: high-accuracy protein contact prediction by coupling precision matrix with deep residual neural networks. Bioinformatics, 35(22), 4647-4655.